Customer loyalty has never been more important – or more competitive.
There are 2 major factors that contribute to this shift. Firstly, the pandemic has been a well-documented catalyst that has transformed consumer behaviour, accelerating the digitalisation of customer experience and levelling the loyalty playing field as customers are drawn to the brands best able to reflect their new outlook and lifestyle.
The second factor relates to technical advancement. Unsurprisingly, blockchain and web3 (read up on Wikipedia) are attracting massive attention from brands both small and large, leading adventurers like Starbucks to explore new token-based loyalty programs as crypto adoption becomes more and more mainstream.
The truth is, the vast majority of businesses value customer loyalty - and trying to capture and sustain it is a journey most are on, albeit in different modes and stages. Today it is almost consensus that data is the most important engine in customer acquisition and engagement. This blog considers when a business can be considered ‘data-ready’ for the implementation of an effective customer loyalty strategy, and the opportunities and pitfalls entailed in building maturity.
I’ll be exploring the theme in the context of an e-commerce retailer specialising in organic, keto and vegan products. Let’s call it Three Body Solver company or TBS for short.
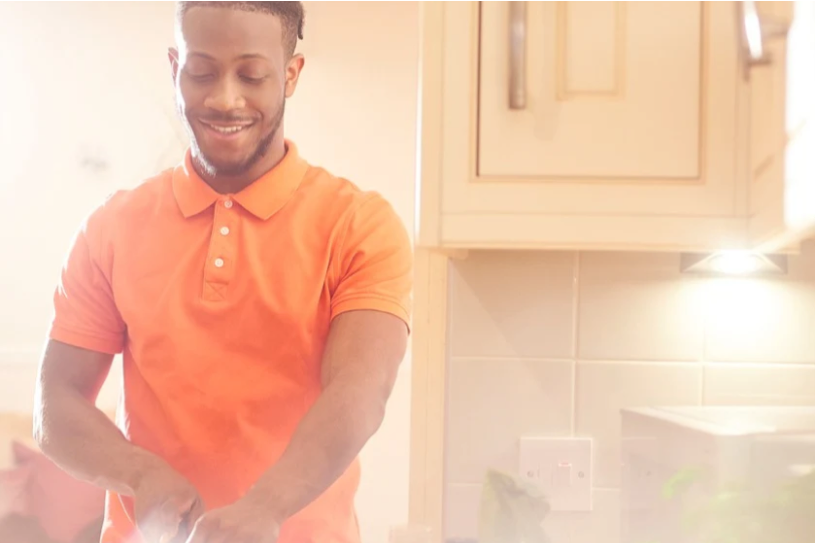
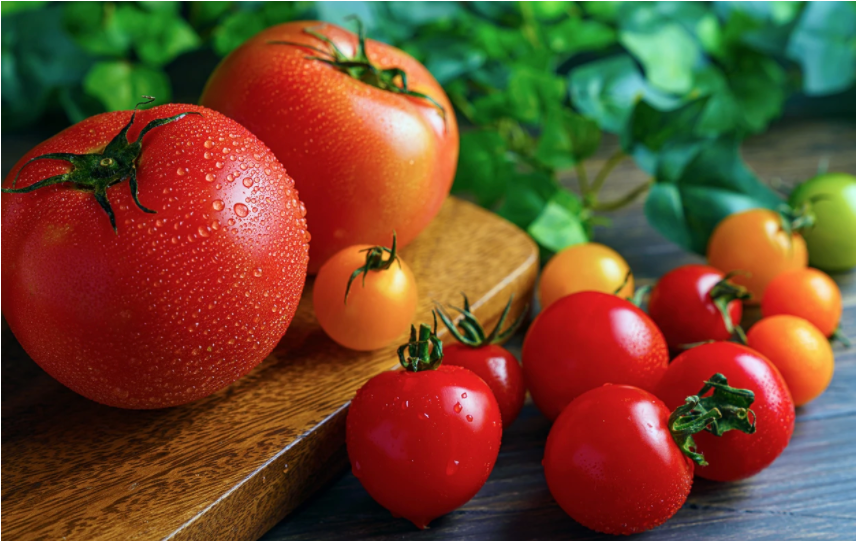
Ambiguous metrics.
Three Body Solver has a strategic goal of increasing customer lifetime value in the next two years by 20%. It made the decision to invest in a data infrastructure and utilises data analytics, data science and machine learning to achieve the growth goal.
Targeting ‘customer lifetime value’ - the historical spend of a customer over time - is a good starting point. However, it’s quite abstract: top line statistics like this don’t reflect or reveal the complex day-to-day activities and interactions between a business and its customers. Instead, we could consider measurable and more specific goals at an operational level. Here’s some potential use cases and metrics that might satisfy the strategic goal.
TBL_When is an organisation data-ready for customer loyalty?
Loyalty goal | Metrics |
---|---|
Reduce 90-day churn rate | Customer retention rate, churn rate, usage frequency |
Increase customer recurring purchase rate | Repeat purchase rate |
Increase monthly loyalty program user activity | Loyalty program registration rate, total revenue, active user counts, referral rate |
True
At Ascent, we always encourage customers to consider loyalty projects only where the metrics of interest can be made available easily. Data that is insufficient quality or incomplete should be deprioritised - sometimes, it is better to reshape the goal or pick a metric that is readily available as a compromise.
Managing a huge volume of data.
TBS have been in business for 7 years; big data is a side effect of its success. Data sources include historical transactions, third-party suppliers, social media and Google Analytics. They started building an omnichannel database (data being generated from every customer interaction) on Microsoft Azure Cloud infrastructure. They fully understand this is a long-term but worthwhile investment for aligning customer experience and appropriate marketing strategies. The data engineering pipeline is the core component. TBS accumulates data in various formats, performing data cleansing and extracting knowledge by running analytics projects.
When managing complex data sets, it is vital to understand how new data aligns with existing reports, dashboards and expert domain knowledge shared across the business. If the gaps are beyond acceptance, it is better to identify the root causes before utilising the data. Conversely, you could switch your approach and tackle low-hanging fruit first, running advanced analytics projects on a slice of customer data of decent quality.
When there is not enough data.
TBS wanted to view the customer journey on their mobile app to understand how customers navigate through the interface, tracking conversion rates at each step. They soon realised certain data within a customer login session was not being captured in their database. In this instance, once the capture mechanism is implemented, you have no option but to wait for the data to accumulate.
In some cases, open-source data or third-party data can be used as either alternative or complementary inputs to proprietary data like historical transactions. A mature data solution is not a bad idea. For example:
Geolocation data from the Office for National Statistics (ONS)
Customer profiling by Experian
Data augmentation, a popular technique mostly in computer vision and NLP areas, is another way to increase data volumes, and we can apply the concept to classical customer data as well. Where data is insufficient in representing an ideal but missing customer segment, as a course of action they can be simulated, and the actual dataset be reverted to for downstream projects.
When the data is poor.
You must have heard the famous ‘garbage in, garbage out’ statement – unfortunately still as true today as it ever was. When data is poor, the customer profiles you derive are likely a misaligned representation of your buying community, which leads to ineffective or incorrect incentives in your interactions.
TBS are considering setting up pop-up stores in order to strengthen their relationship with customers in a physical setting. Where could be the best locations and neighbourhoods for these temporary shops or stalls? What products should they supply to best attract local fans? In order to make insightful decisions, they wanted to understand the relationship between customer locations and purchase behaviour from data.
Once data was pulled for analysing, they immediately noticed that only 72% of customers had addresses in their database. It turned out that some guest transactions went through a third-party website where their addresses get registered for delivery. While waiting for the missing data to be filled in, they focused on customers with known addresses.
Next, they wanted to join public geographic and socioeconomic information such as population, region and GDP per head based on postcode. Many postcodes were problematic as they were not in expected format or not valid at all, so after fixing the issue in historical data, their UI team replaces the free text box with a postcode lookup.
In this case, they identified the root cause of the problem, and came up with ways to work with imperfect data. It is worth highlighting the importance of data engineering. Our goal is not only customer data of good quality but also a reusable data pipeline – how data is transformed and processed. It may require iterations to achieve a data pipeline that meets a best practice standard. A positive action would be to assess the impact of data quality and prioritise those important battles.
Resource allocation.
The more engaged your customers are, the more data you collect. The ability to leverage this data to delight customers creates competitive edge. But how much have you invested in the capability to surface this data? How do you allocate headcount of a data team? Do you invest in a customer insight software or an application that automatically spits out the expected output once data is plugged in? Or hire more data talent and integrate it into customer loyalty functions? (not as easy as it sounds…).
The benefit of tooling is that people with limited relevant knowledge and skills in the organisation could generate seemingly useful insights immediately. On the downside, it can require a sizable investment and might only work like a charm when your data is the perfect fit (unlikely!). Equally, it cannot replace your effort on experiments and tests in creating the most effective rewards. So we suggest cautious evaluation before purchasing an ‘automatic’ solution.
Another option is to invest in people. Shared goals and a shared vision allow an integration of business teams and data talent ensuring successful data analytics projects. Though it takes time and resource for any growing retailer company like TBS to build up teams, hire the best individuals and improve team dynamics to realise value.
Seeking external support from consultants may be a sensible compromise to enhance an existing team. In the case of TBS, they were able to focus on the supply chain and collaborate with an outside group of data experts on combing through customer data. While working closely with them, TBS were able to figure out what qualities were required and which candidates match up with the company’s vision, as well as upskilling their current teams. The question of who to hire gets solved naturally at a later stage.
Data privacy considerations.
Among companies who are fined for a GDPR violation, a most frequent cause is non-compliance with lawful basis for data processing of customer information such as age, gender and location. As such, customers should be informed explicitly about how their data is used and for what purpose. We suggest that you review your privacy policy carefully before embarking on any customer loyalty project.
So, am I ready?
While TBS are just beginning their journey harnessing the power of data, their customers are heading back with smiley faces and 5-star reviews again and again. It’s all about mindset – if you are prepared for a long term commitment, you value the skills and capability required to make your loyalty projects a success and you understand the (potential) value of your data – go go go! It doesn’t need to be a ‘big bang’ project – you can start tomorrow, prove value quickly with even the smallest of incremental improvements and accelerate your investment organically as you start to see your efforts rewarded.
Got a similar challenge or just keen to find out more about our interesting use cases? Our award-winning data science team is on hand to help!