It’s International Women’s Day and we couldn’t be happier to support the campaign to break bias, call out inequality and promote the visibility of the achievements of anyone who identifies as a woman. Not only are women underrepresented in the field of data science and analytics, but there are also fundamental flaws in the collection, management and modelling of data that perpetuate gender imbalance and inequality.
Even in 2022, women are still dealing with the ripple effects of historical statistical exclusion: their accomplishments, needs and experiences are less well-documented, analysed or ingrained. This has made women less visible and more ‘other’ as male data has become the ’neutral’ default, a subject expertly covered in Invisible Women: Exposing data bias in a world designed for men by Caroline Criado Perez. In modern analytic practices it is also a widely acknowledged and well-researched problem that automation often surfaces the human bias unconsciously designed into algorithms, and Data Science models using AI and ML can amplify or intensify any existing biases with potentially harmful results.
Where does bias come from?
Before we consider the practical steps we can take to address the issue, let’s look at how and where bias sneaks in. Ava Yang, one of Ascent’s Senior Data Scientists gives us a typical example with potentially serious consequences.
“Consider a medical program that is used for early identification and treatment of a certain cancer. The data that feeds into an AI/ML model may contain biases that originate from a point before the data was collected. Bias flaws in the collection approach (like lack of researcher diversity, the nature of the data chosen for collection, subject group composition or even the choice of language and format of questions) could ultimately lead to higher admission rates into a medical treatment program of one group over another.”
There’s many examples where latent gender bias actively becomes dangerous for women, like in the (mis)diagnosis of heart attacks (medical training typically focuses on male symptoms, which are different from women’s; diagnostic intelligence tools are trained on male-oriented datasets). Or the product testing of seatbelts, traditionally optimised to protect a 50th percentile male body as representative of an average human.
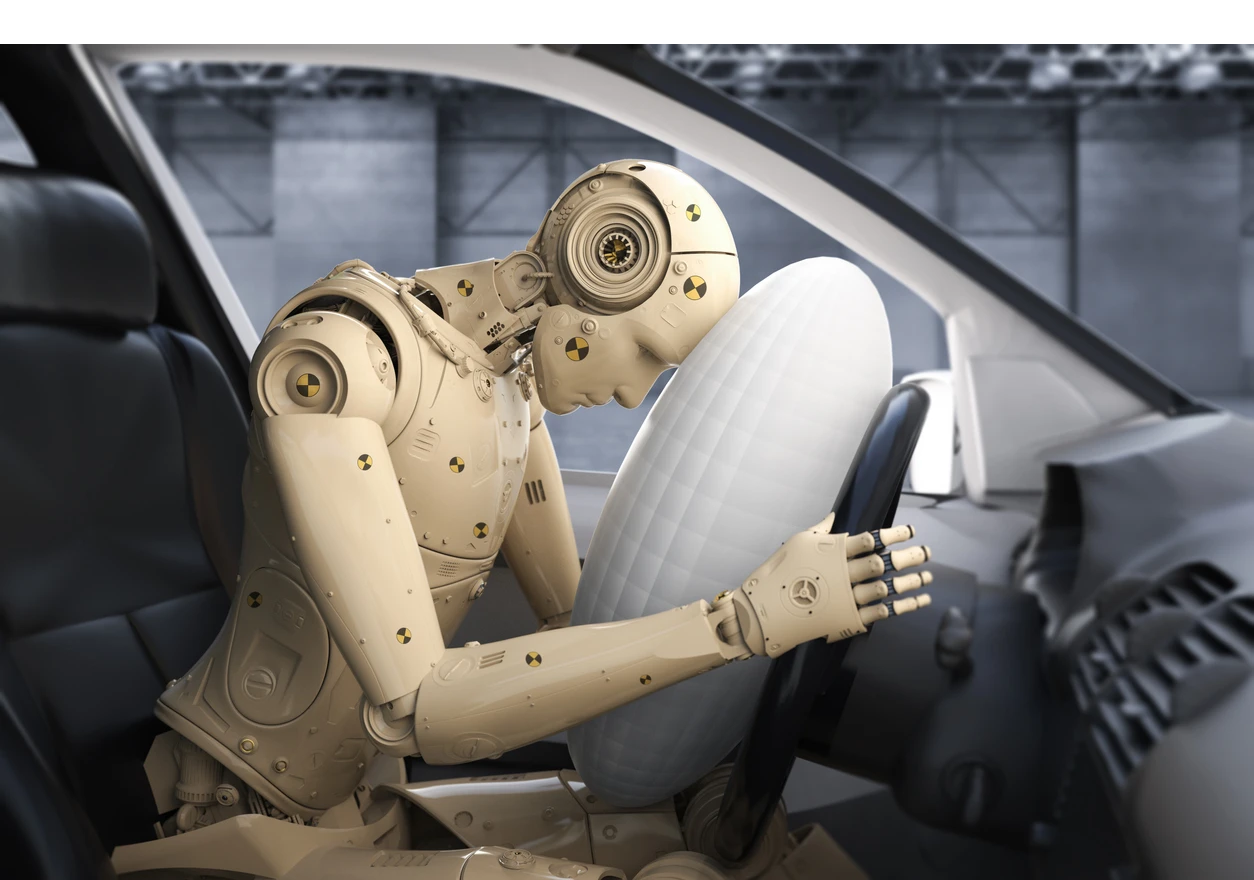
Bias mitigation.
So who is on the hook for a) identifying and b) minimising the instances and impact of data bias? Data scientists are responsible for monitoring the data pipeline, processing the data and interpreting the results. Understanding models and ensuring they are truly reflective of the real world is also a key part of the job. “In the earlier scenario of the cancer treatment program”, said Ava, “practitioners would need to closely measure disparity in predictions and prediction performance amongst different characteristics of the population (age, gender, race) for fair representation. If disparity is confirmed, further human intervention is necessary to mitigate bias, considering a series of questions like these:
Is the bias in predictions caused by misrepresentation in the Machine Learning model training data where for example, female observations are underrepresented?
When comparing prediction performance in error analysis (a post-modelling process), is the model performance worse for female or male?
Can we calibrate results so the medical admission rates are more balanced among genders to fix issues in the historical decision-making process?
Between models with varying levels of disparity, can we opt for a model with a lower disparity (still with an acceptable level of accuracy)?"
There are a few steps operationally that organisations can take to help mitigate bias when working with data and tech, but they depend on an increased awareness of our own biases and our ability to challenge and rebuild. We spoke to Ascent’s Principal Data Consultant Branka Subotić, who has spent 20 years helping companies understand how to harness their data to drive business value.
“Business leaders need to live and breathe diversity in the way their organisations are set up and run; not only in the way they recruit and search for talent but also across all processes, including the management of data,” advises Branka. She considers some practical ways to help manage data bias at an organisational level:
Audit where biases may exist across the organisation, with particular emphasis on recruitment and training
Increase diversity in your teams to make your intelligence less subjective and more robust because of its collection and analysis approach
In your recruitment processes, ensure that the interviewing panel receive CVs that are stripped of all personal data
Create a pool of interview questions and make sure these are reviewed by your Equality Network for unintended bias (or seek support from various charities that exist out there, like Business Disability Forum
Consider 3rd party auditing to highlight and eliminate any underlying biases.
The road to better data.
The steps we take today toward eradicating data bias won’t pay off immediately as ‘better’, more representative data takes time to accumulate in new structures and frameworks, but the opportunity to challenge, optimise and strengthen our approach is in front of us now.
Happy International Women’s Day to absolutely everybody.
#breakthebias #internationalwomensday